KYC Article
Neobanks: How AI can turbocharge your KYC process
Artificial intelligence is changing the neobanking landscape, with machine learning ready to power up your identity verification process, boost your conversion rate and accelerate your ROI.
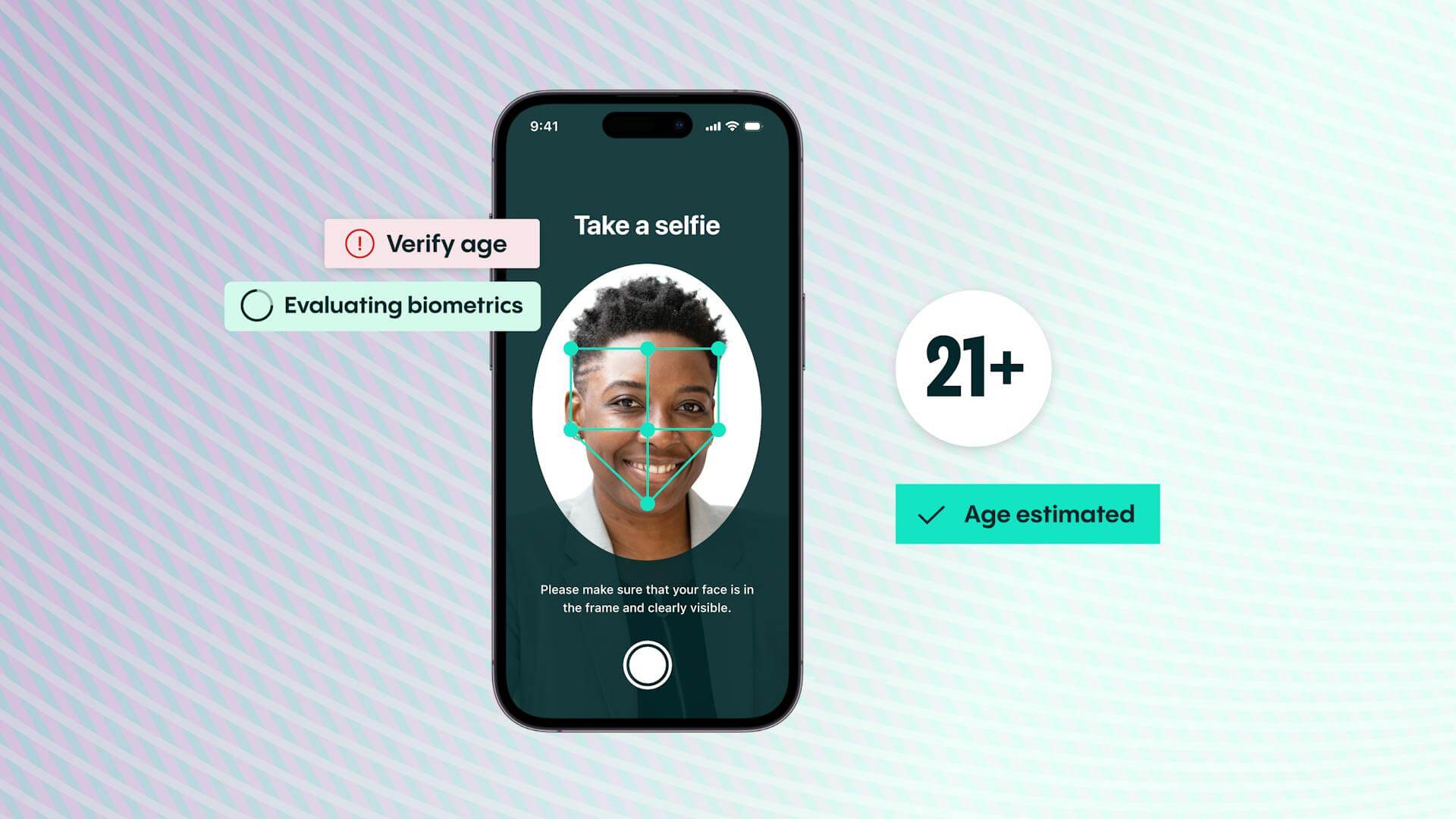
Maximize conversions while minimizing risk
In traditional banking, a financial institution is required to prevent financial crimes and illegal activities, such as terrorism financing, by implementing practices to meet know your customer (KYC) requirements.KYC compliance involves verifying customers before granting access to everyday services like opening bank accounts, getting debit or credit cards. In financial services, the need remains to verify the identity of would-be customers, but with the growth of the digital bank worldwide, institutions are looking for more innovative solutions to complete enhanced due diligence (EDD), customer due diligence (CDD), and other compliance measures.
Of course, alongside these developments, we are also seeing the rapid advancement of Artificial intelligence (AI) in the form of machine learning – the application of which promises to be transformative for many sectors. However, as well as being transformative in a positive sense – delivering automation, efficiency, increased productivity and cost reduction – it also brings enhanced risk. For financial services firms, that includes heightened security and fraud risks – and AI is making it easier for individuals to carry out identity fraud.
Neobanking is no exception. By analyzing huge volumes of digital data, automated decision engines can recognize patterns and progressively improve the speed and accuracy of processes. This kind of machine learning is already being used with great success by neobanks, both to improve fraud prevention and to deliver a better user experience. But they, too, also face the negative risks posed.
What exactly are neobanks and how do they differ?
Neobanks, sometimes referred to as “challenger banks,” are fintech firms that offer apps, software and other technologies to streamline online customer experience (mobile / online banking) . These fintechs generally specialize in particular financial products, like checking and savings accounts. They also tend to be more agile and transparent than their megabank counterparts, even though many of them partner with such institutions to ensure their financial products.
In the U.S., these fintechs are more commonly referred to as neobanks. The term “challenger bank” was first popularized in the U.K. to refer to a number of fintech banking startups that emerged in the wake of the 2007-2009 financial crisis.
Do neobanks face greater risks?
Neobanks do come with certain risks that potential customers should be aware of:
1. Regulatory and legal risks
- Regulatory environment: neobanks often operate under different regulatory frameworks compared to traditional banks. Some may not have the same level of regulatory oversight, which can affect their stability and customer protections.
- Licensing: some neobanks operate under partnerships with traditional banks, while others may have their own banking licenses. The level of protection can vary depending on the licensing arrangements.
2. Financial stability
- Profitability: many neobanks are not yet profitable and rely on venture capital funding. This can pose risks if they are unable to sustain operations or secure additional funding.
- Resilience to economic downturns: as newer institutions, neobanks might not have the same financial resilience as established banks, potentially making them more vulnerable during economic downturns.
3. Technology and security risks
- Cybersecurity: as digital-only platforms, neobanks are highly dependent on technology. They must maintain robust cybersecurity measures to protect against hacking, fraud, and data breaches.
- Technical issues: outages or technical issues can disrupt access to funds and services, causing inconvenience and potential financial losses for customers.
4. Customer support and service
- Limited physical presence: the absence of physical branches can be a drawback for customers who prefer face-to-face interactions for complex issues or concerns.
- Customer service quality: the quality and availability of customer support can vary, with some customers experiencing difficulties in resolving issues promptly.
5. Deposit insurance and fund protection
- Deposit insurance: it’s essential to verify whether deposits are insured and to what extent. In the U.S., for example, traditional banks are typically insured by the FDIC, but this may not be the case for all neobanks.
- Funds protection: understanding how your funds are protected in the event of the neobank’s failure is crucial. Some neobanks partner with traditional banks for deposit insurance, while others may have different arrangements.
6. Trust and reputation
- Reputation: as newer entities, neobanks may not have established a long track record of trust and reliability. Researching customer reviews and the bank’s history can provide insights into their reputation.
Fast decisions
A 98% check automation rate gets customers through in about 6 seconds.
Simple experience
Real-time end user feedback and fewer steps gets 95% of users through on the first try.
Document coverage
An unmatched 12K+, and growing, government-issued IDs are covered.
More conversions
Up to 30% more customer conversions with superior accuracy and user experience.
Better fraud detection
Veriff’s data-driven fraud detection is consistent, auditable, and reliably detects fraudulent forms of identification.
Scalability embedded
Veriff’s POA can grow with your company’s needs and keep up with times of increased user demand.
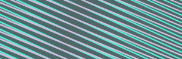
Talk to us
Our identity verification experts are ready to help build you a solution that gets more honest customers onboarded faster, and more securely, than ever before
Speak with the experts at Veriff
If you run a financial institution, then having proper anti-money laundering customer due diligence processes in place is vital. Thankfully, our IDV & AML Screening solution can help you fight financial crime and show regulators that you take financial crime and compliance seriously.
Veriff’s Proof of Address product has been bolstered with additional data extraction capabilities to work seamlessly alongside our product ecosystem. The updates to POA will further reduce user friction, lower costs, and continue to comply with regulators. Ensure your customers aren’t waiting hours, or even days, for approval.
If you’d like to hear more about how our solution can help you, then talk to our compliance experts today.
Improving accuracy
IDV is a critical component in the KYC process, mandated by regulators to combat money laundering. The best IDV systems compare live biometric data from a selfie with stored biometric data to authenticate customer sessions. By incorporating machine learning algorithms programmed to identify patterns into this kind of automated IDV solution, the data collected can be used to adjust and improve the accuracy of its decision-making over time.
Because machine learning makes predictions based on previous experience, including previous fraud schemes, novelty is a potential blind spot for the technology. The larger and more current the dataset, therefore, the lower the risk that an automated decision engine will make a mistake. For identity verification, for example, access to a dataset that includes plentiful biometric data for a range of ethnicities and ages is important in minimizing potential bias. In addition, cross-comparing verification sessions based on device, network and customer behavior can provide a greater richness and variety to the data a decision engine uses to learn and improve its decision making.
By analyzing huge volumes of digital data, automated decision engines can recognize patterns and progressively improve the speed and accuracy of processes. This kind of machine learning is already being used with great success by neobanks – and by Veriff.
IDV is a critical component in the KYC process, mandated by regulators to combat money laundering. The best IDV systems compare live biometric data from a selfie with stored biometric data to authenticate customer sessions. By incorporating machine learning algorithms programmed to identify patterns into this kind of automated IDV solution, the data collected can be used to adjust and improve the accuracy of its decision-making over time.
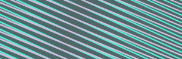
Get more details
Discover more about how IDV is powering Neobank growth and customer acquisition.