Blog Post
How Veriff is building trust through tackling bias
Bias, present in machines and people, poses a risk to honest users looking to get verified securely, seamlessly and fairly. Discover the steps Veriff is taking to reduce bias to create an infrastructure for trust online.
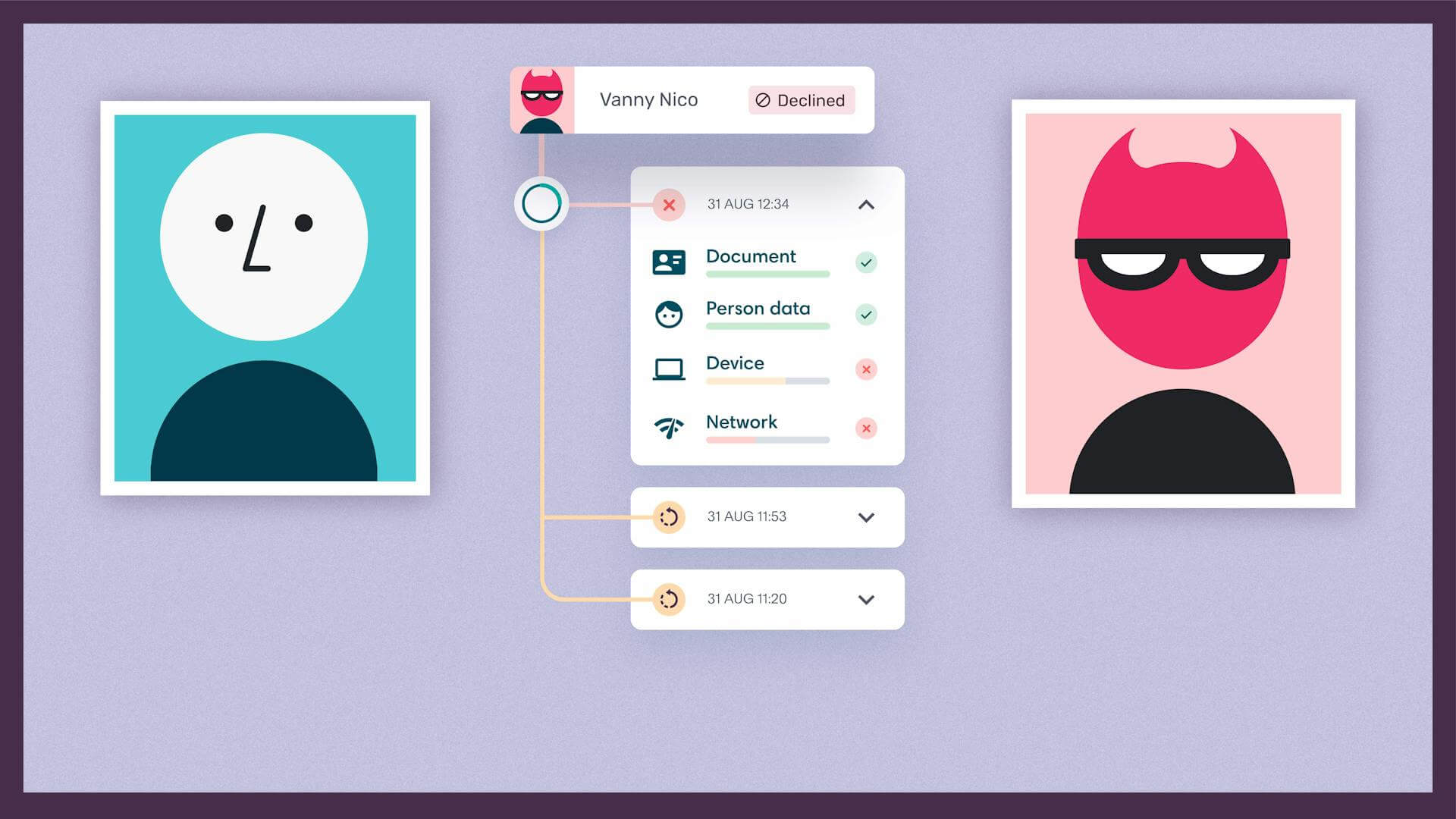
At Veriff, we’re proud of our team’s diversity, with our European, British, and American offices staffed by professionals drawn from over 40 different countries. It’s vital that we encompass a range of different backgrounds and perspectives to achieve our mission: creating a platform which allows honest users from across the world to access online services quickly, securely, and seamlessly.
We go to great lengths to find top talent to enhance both our organizational culture and our offering to users. However, Veriff doesn’t exist in a vacuum; according to recent research, the technology sector in Estonia (our corporate headquarters) has three male professionals for every one female professional.
These disparities can be understood as a result of bias, where people have a disproportionate favorability or unfavorability towards other people or things, including traits like ethnicity, age, or gender. Many people are aware of some of their personal biases, but unconscious bias occurs without awareness of their own prejudice.
Human bias is formed from a number of different factors, such as upbringing, culture, and environment. Put bluntly, all humans have some form of bias; a long-standing promise of innovative technology has been the idea that automation can allow for greater objectivity and clarity, with the goal of fairer outcomes for users. However, recent trends have proven that bias can exist in innovative technology in a number of ways.
For instance, take the real-life example of an abandoned automated recruitment tool used by a major online retailer. The recruitment tool’s goal was to screen CVs and find top candidates for technical positions — but the tool picked only males. Many of the world’s largest companies have predominantly male engineering workforces, and these workforces are where much of the data for these tools are pulled, so the automated system learned that males were preferable for the roles.
We can see that a great deal of users face unfair treatment when using an automated service. Veriff primarily utilizes automation to validate users, but also employs human specialists. To eliminate as much human bias as possible, we control the verification decision by having historical verification use cases mapped and larger processes agreed upon. As part of QA, we guarantee control by instituting an alignment test that controls how different people answer different questions and makes sure the answers are aligned and consistent.
We monitor biases as part of our overall QA process because of the risk of mistakes. QA’s job is to review a defined percentage of verification sessions daily with a goal of detecting mistakes. The sessions sent for review are selected randomly from all sessions, but there are controls in place to receive a representative number of sessions from users based on their country, document types, clients, and if the session was completed by automation or by specialists.
This review process assures that we are able to detect mistakes in our decisions quickly. The output from the quality assurance process is tracked on a weekly basis as a key internal KPI. The issues found during the QA process are then raised by relevant teams, who will prioritize them and take relevant actions to avoid future mistakes.
Automatic decision bias depends mostly on the data used for training. Veriff trains models with real live data from clients who have granted permission. The use of live data assures that we have good coverage of a range of documented biases in the training set. We work so that we are covering different use cases with the data and control it periodically. We are ensuring that we do not overfit different cases by controlling how much data we have for a case in a training set.
Another risk that needs to be addressed and tackled is document bias. Document bias occurs due to the reality that identity documents vary greatly between different countries, with factors including language, security features, photograph quality, and more. However, Veriff works to ensure that this doesn’t become a barrier to validating honest users.
For instance, we’ve built a market-leading specimen database, able to process over 12,000 document types from 230 countries. Our team regularly refreshes the database as new types of I.D. are issued and existing document types are upgraded.
We are constantly updating our processes based on best practices, new research, and insights from our team. Having an international, multi-ethnic team is invaluable to eliminating bias within our automated and human operations. Team members will be able to address each other’s unconscious biases and draw attention to a range of user needs, while a focus on neurodiversity helps to innovate processes. As Veriff continues to expand, we look forward to continuing to promote diversity as a core value and one that benefits our user base.
Another way Veriff is working to reduce bias is by building an in-house facial recognition system. By creating our own model, we are able to train it using our own data and we can have the annotations done by our own teams. We are looking to create a model which is more accurate, intuitive for the user and less likely to produce mistakes.
To summarize, Veriff seeks to utilize the best of what technology has to offer as well as the option of human QA. That said, we need to strike the right balance of accuracy and automation; if we solely pursue the former, we may produce more verifications, but it’ll take longer, be less user friendly, and difficult to scale. If we just went for the latter, we risk more mistakenly failed verifications and a lack of review over potentially developing biases. Bias may always be a fact of life in humans and machines, but at Veriff we’re focused on reducing it as much as possible to benefit our users and achieve our mission.